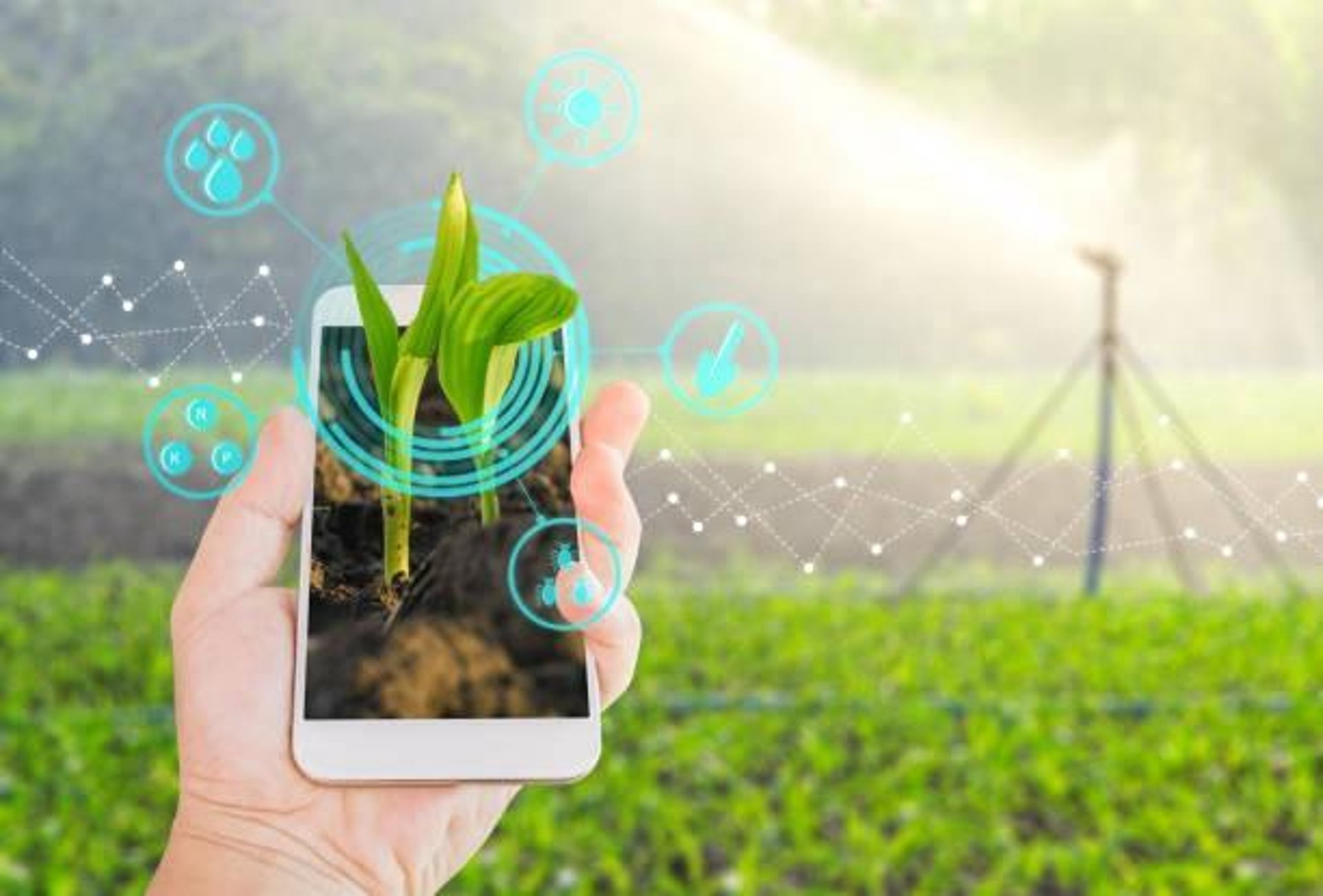
Smart Agriculture
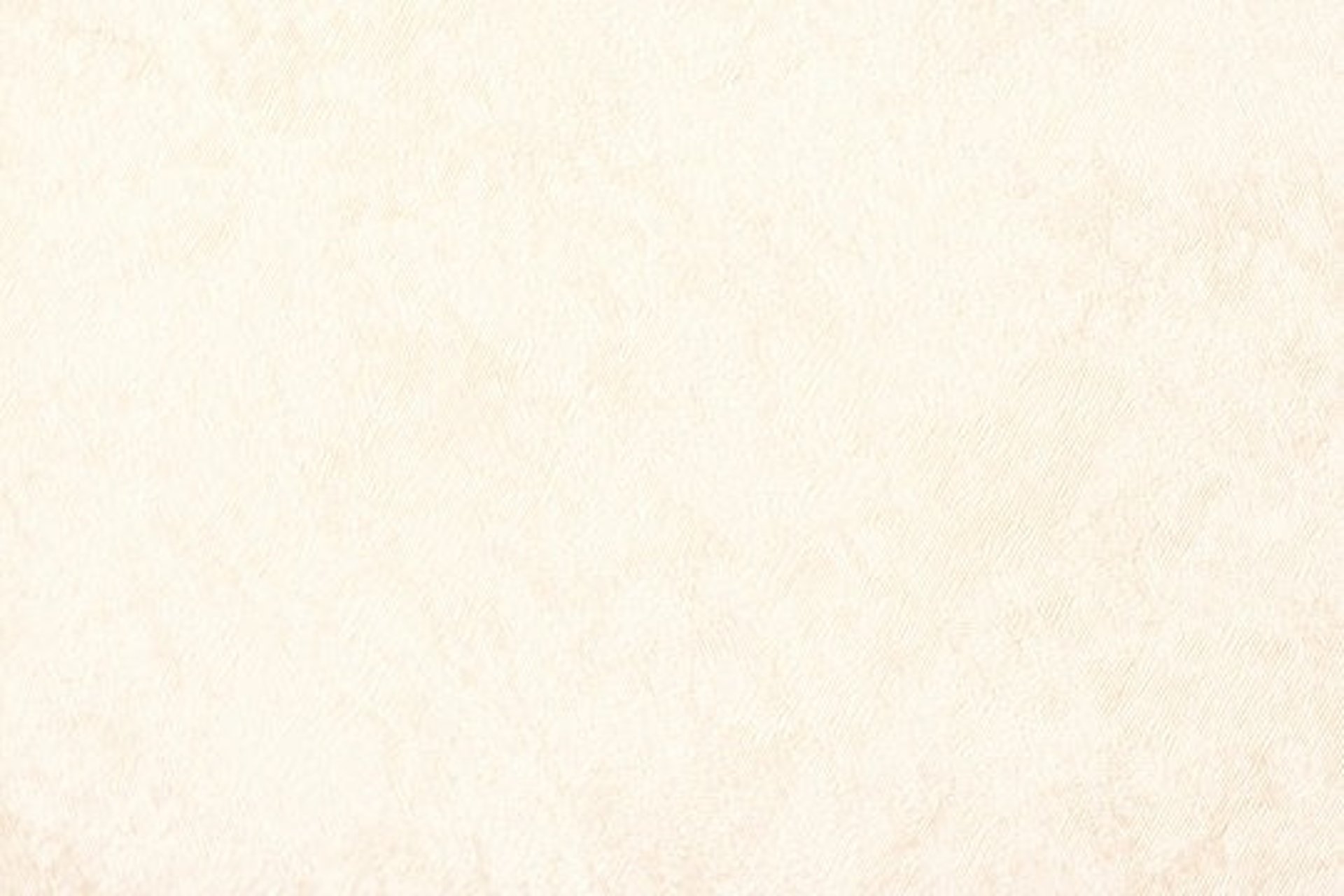
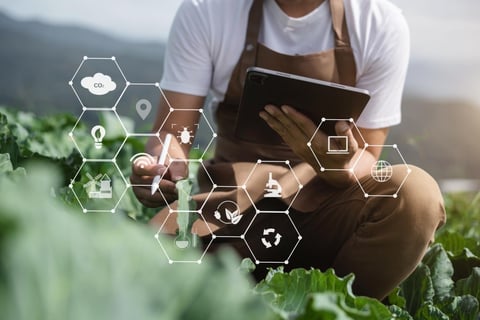
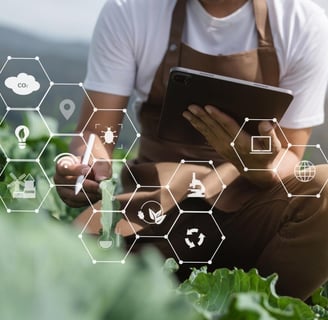
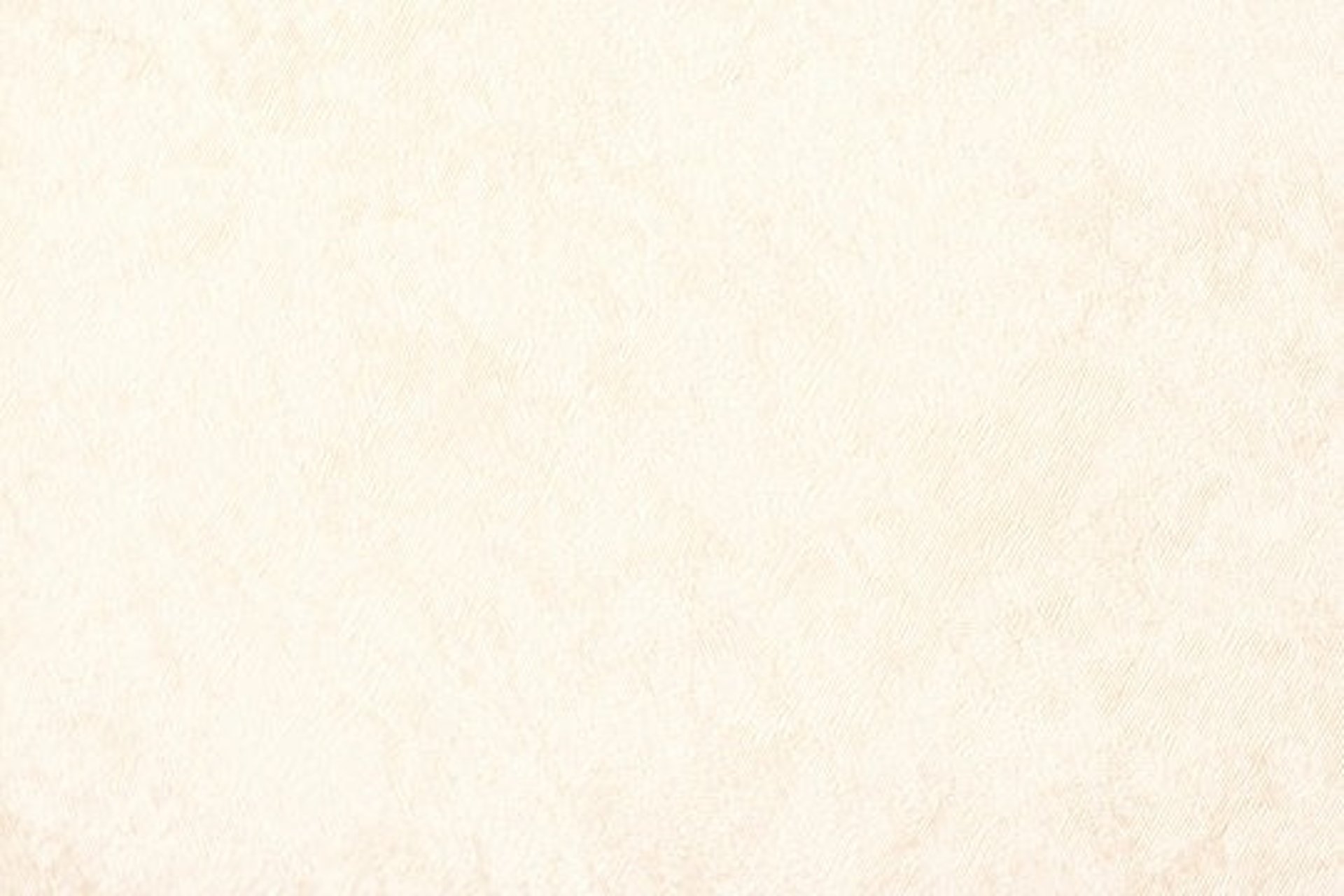
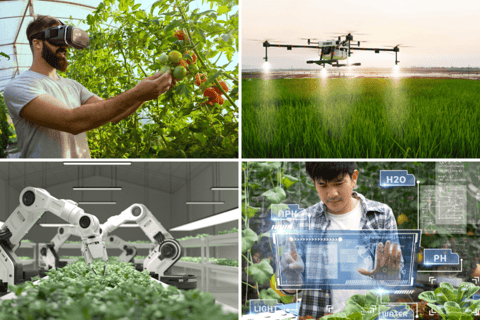
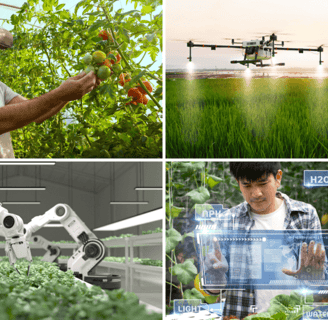
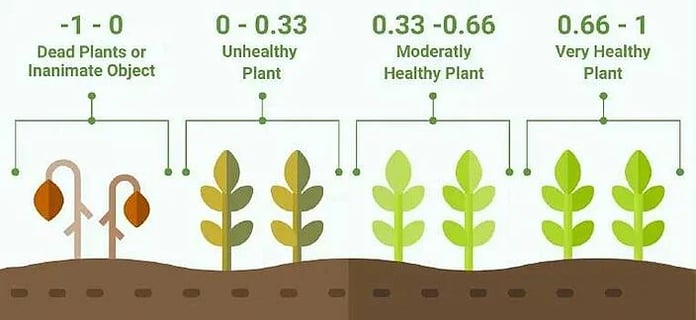
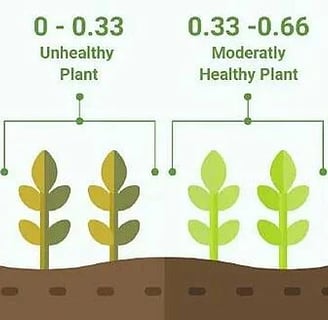
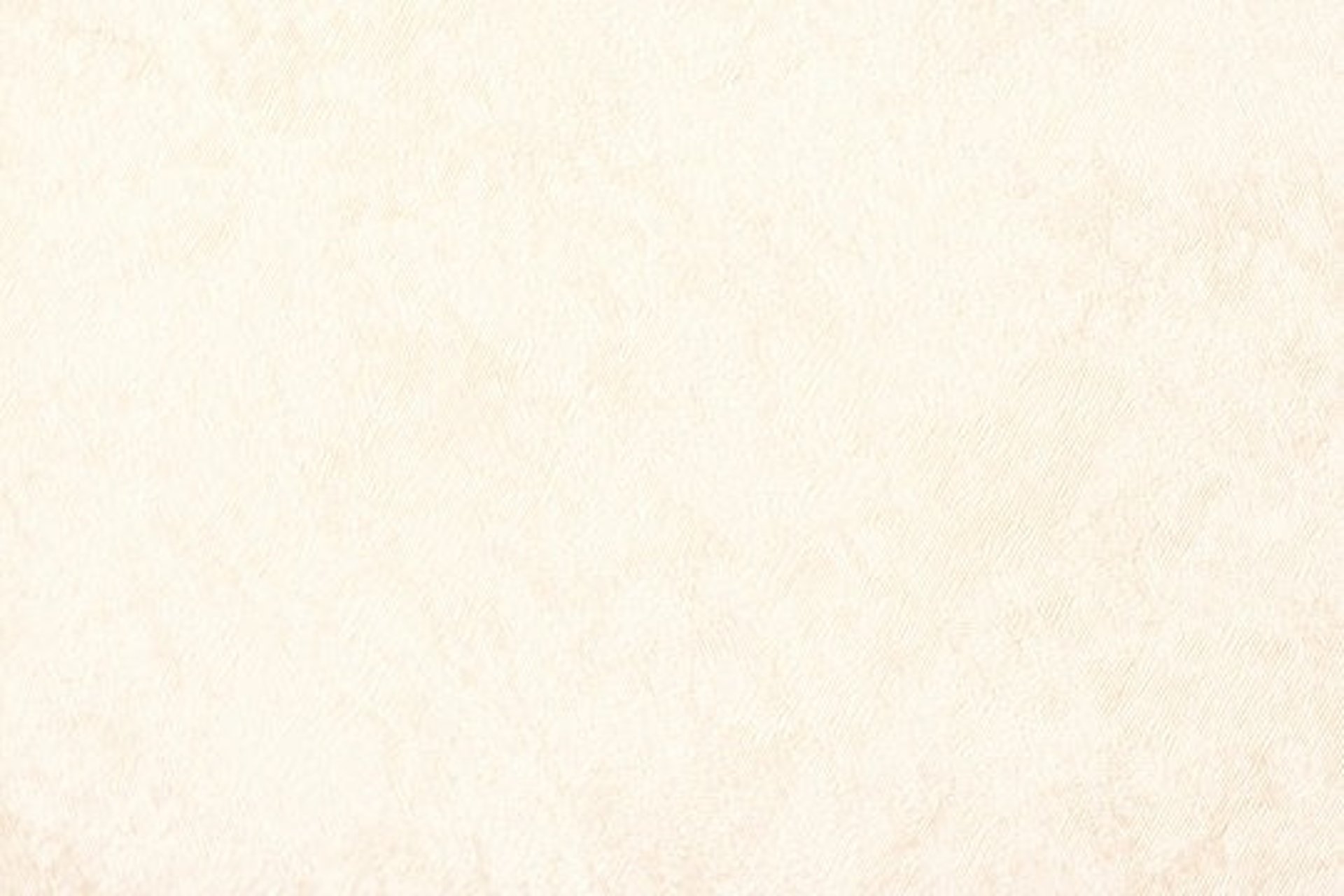
Analysis at Nams Lanka Farm
The study area is located in Sri Lanka and is shown in below Figure 1
The study area is located in Sri Lanka and is shown in below Figure 2
The study analyzed small agricultural subplots shown in Figure 1 for NDVI forecasting for different vegetation types. Using LSTM models calibrated with past data, 2-3 NDVI 2-3 weeks a head can be predicted.
Models calibrated with different irrigation supply provides a mechanism for estimating irrigation requirement.
SCL (Scene Classification) data are used to filter out pixels that are covered by clouds or shadows
Missing data were interpolated based on the surrounding values.
Other satellite data, such as Landsat-8 and MODIS, will be used to compensate for cloud cover in Sentinel-2 data
Efficient methods for NDVI forecasting for larger areas based on clustering of similar agricultural fields will be developed